Contents
- 1 Google DeepMind Revolutionizing Weather Prediction
- 2 Unveiling the Triumph
- 3 Evolving Weather Simulations
- 4 Training for Precision
- 5 Advancements and Future Prospects
- 6 Recognition and Excitement
- 7 Google DeepMind Ongoing Challenges in Forecasting
- 8 Climate and Forecasting Dilemma
- 9 Google DeepMind Strides and Future Directions
Google DeepMind AI unit achieved a remarkable feat, surpassing Europe’s foremost weather center in predicting 90% of atmospheric measures. The machine learning algorithms, after analyzing extensive decades-old weather data, showcased unparalleled accuracy compared to Europe’s top weather center.
Google DeepMind Revolutionizing Weather Prediction
DeepMind’s AI approach involves employing graph neural networks (GNNs) to model fluid behavior, leveraging its expertise in machine learning. This system, despite requiring heavy-duty training, yields a lightweight final model. GNNs represent data as interconnected nodes, simulating atmospheric conditions globally, and predicting their interactions over time.
In September, Google’s DeepMind AI unit in London closely monitored Hurricane Lee’s trajectory, approximately 10 days before its projected landfall. While official forecasts vacillated on the storm’s path, DeepMind’s experimental software, GraphCast, confidently pinpointed a more northerly landfall. Researchers, including scientist Rémi Lam, were captivated by the accuracy of their prognosis.
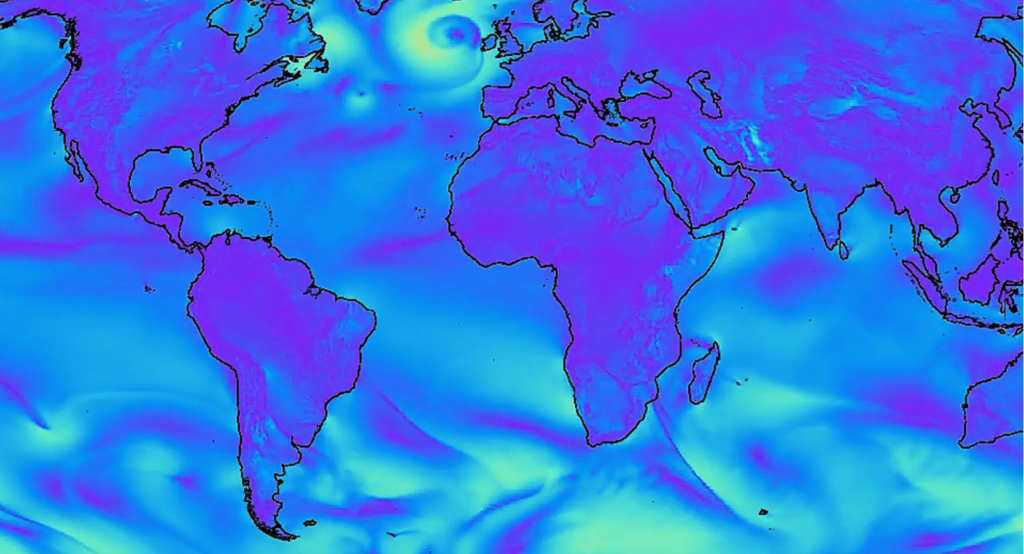
A week and a half later, Hurricane Lee made landfall precisely where DeepMind’s software predicted—Long Island, Nova Scotia. Thus, considerably distant from major population hubs. This success mirrors a groundbreaking period for AI-powered weather models, signaling an impending transformation in the field, a sentiment echoed by seasoned forecasters.
Unveiling the Triumph
Presenting substantiated evidence of its potential, Google published a peer-reviewed paper in Science. DeepMind’s model outperformed forecasts from the European Centre for Medium-Range Weather Forecasting (ECMWF) across 90% of over 1,300 atmospheric variables, including humidity and temperature. Notably, the efficiency of the DeepMind model stood out as it could generate forecasts within a minute on a standard laptop, while conventional models necessitate substantial supercomputing resources.
Read More: Humane AI Pin: A Revolutionary OpenAI-Powered Wearable
Evolving Weather Simulations
Conventional weather simulations rely on replicating atmospheric physics and assimilating detailed weather observations from numerous sensors and satellites. While advancements have enhanced their accuracy, these models remain laborious, often taking hours to compute on potent servers at major weather centers.
Training for Precision
Training DeepMind’s networks involved 39 years’ worth of ECMWF observations to accurately predict evolving weather conditions over six-hour intervals. By teaching the software to anticipate atmospheric pattern shifts, these forecasts extend up to a week, showcasing the potential of AI in revolutionizing global weather prediction.
Advancements and Future Prospects
Rémi Lam and Peter Battaglia, researchers behind DeepMind’s forecasting model, envision their current success as a stepping stone. The model’s versatility prompts plans for customized versions, targeting improved performance in specific weather conditions like precipitation, extreme heat, hurricane tracks, or for more detailed regional forecasts. Google explores integrating GraphCast into its products, a move evident in recent AI model additions to its mobile weather forecasts.
Recognition and Excitement
Matthew Chantry, from ECMWF, acknowledges GraphCast as the foremost AI contender, projecting its continued incremental improvement. He highlights the software’s unique offering of precipitation forecasts, a challenging feat due to the finer resolution required for predicting rainfall compared to available training data.
Read More: GPT-4 Turbo: OpenAI DevDay Reveals An Ultimate AI Model
Google DeepMind Ongoing Challenges in Forecasting
Despite Google’s AI success, challenges persist in weather forecasting. Ensemble forecasts, detailing multiple potential outcomes and probabilities for major weather events like hurricanes, remain beyond the current AI model’s scope.
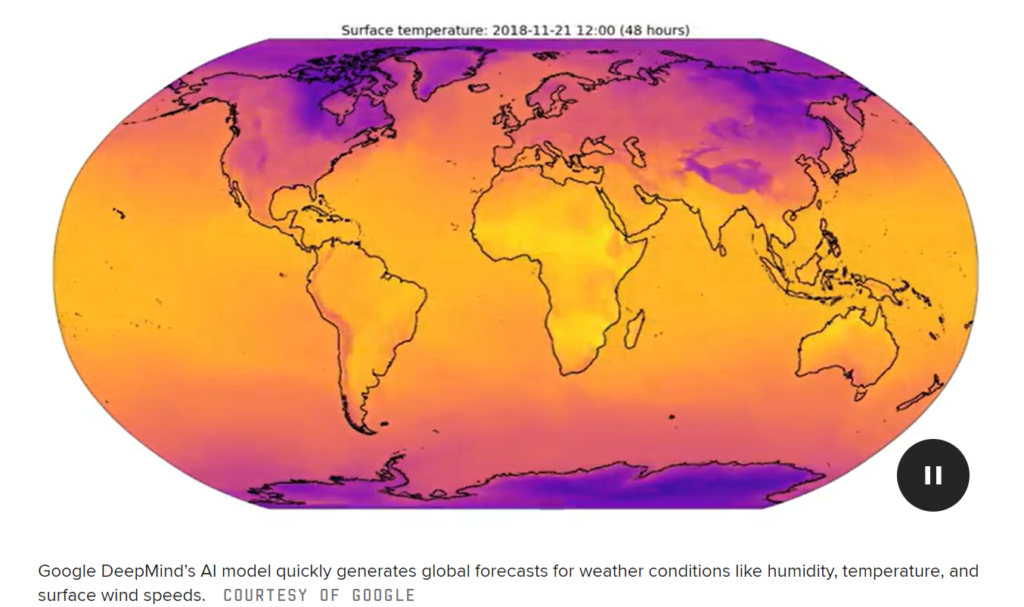
AI models exhibit a tendency to underestimate the strength of significant events such as Category 5 storms. This could stem from their inclination toward average weather predictions, being cautious about forecasting extreme scenarios. Additionally, GraphCast fell short in predicting stratospheric conditions compared to ECMWF’s forecasts, though the reasons remain unclear.
Climate and Forecasting Dilemma
Training AI models on historical data raises concerns about their adaptability to future weather patterns. While traditional models, grounded in physics laws, exhibit robustness to climate changes, AI models might struggle if future weather diverges significantly from the past.
Google DeepMind Strides and Future Directions
DeepMind’s system’s proficiency in predicting varied weather systems despite limited training data suggests an internalized understanding of atmospheric physics. Emphasizing the need for current data in training, Peter Battaglia underscores the importance of updating models to align with evolving weather patterns.